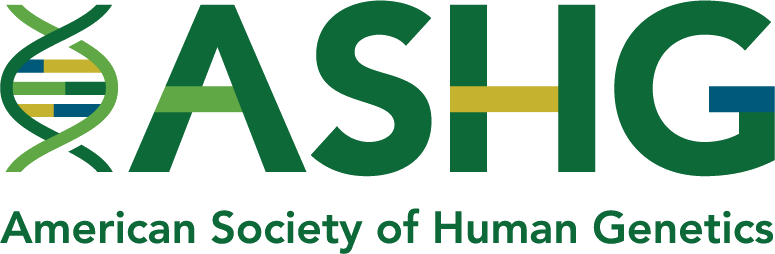
Stability of Polygenic Scores Across Discovery GWAS
-
Register
- Regular Member - Free!
- Early Career Member - Free!
- Resident/Clinical Fellow Member - Free!
- Postdoctoral Fellow Member - Free!
- Graduate Student Member - Free!
- Undergraduate Student Member - Free!
- Emeritus Member - Free!
- Life Member - Free!
- Nonmember - $20
- Trainee Member - Free!
Laura Schultz, PhD, will discuss the surprising lack of consistency between polygenic scores (PGS) computed from subsequent generations of consortia-produced discovery GWAS for height, post-traumatic stress disorder, and type-2 diabetes. For a given trait, PGS are only modestly correlated at the level of the individual subject, and different individuals are identified at the upper quantiles of the PGS distributions.
Overview of Presentation
- PGS calculated from pairs of same-ancestry discovery GWAS show only modest correlation with each other despite there being near-perfect genetic correlation between the discovery GWAS.
- A series of height GWAS in the UK Biobank suggests that correlation between PGS is strongly dependent on the extent of sample overlap between the discovery GWAS.
- Focusing on the upper tail of the PGS distribution, different discovery GWAS do not consistently identify the same individuals. The degree of overlap decreases sharply as higher quantiles, less heritable traits, and different-ancestry GWAS are considered.
- PGS computed from different discovery GWAS have only modest correlation at the individual level, underscoring the need to proceed cautiously with integrating PGS into precision medicine applications.
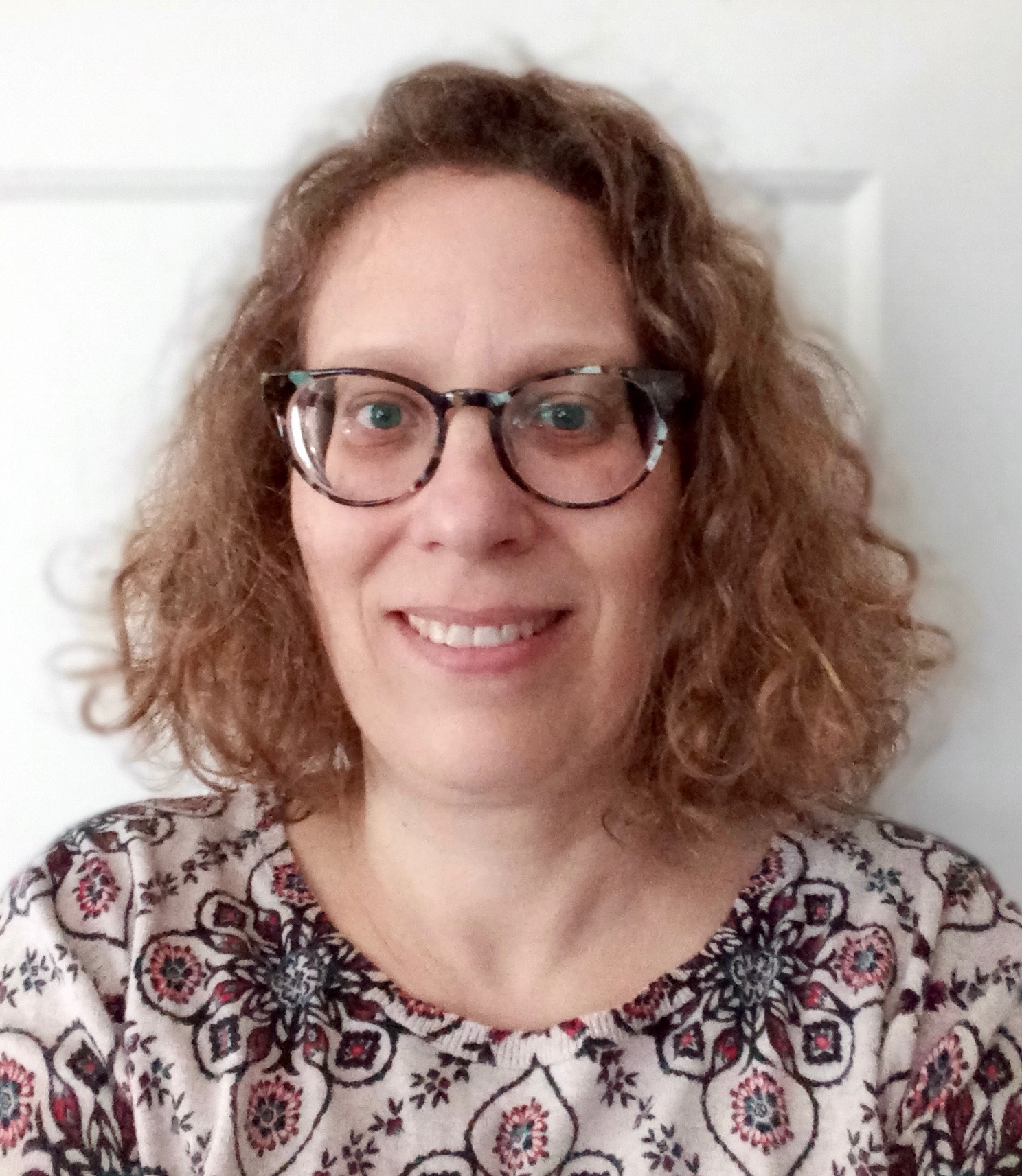
Laura Schultz
Data Scientist
Department of Biomedical and Health Informatics at the Children's Hospital of Philadelphia (CHOP)
Dr. Laura Schultz is a Data Scientist in the Department of Biomedical and Health Informatics at the Children's Hospital of Philadelphia (CHOP) who is also affiliated with the Lifespan Brain Institute (LiBI) at CHOP and the University of Pennsylvania. She earned her A.B. in psychobiology from Ripon College and her Ph.D. in psychology and neuroscience from Princeton University, and she completed a postdoctoral fellowship in computational neurobiology at the Salk Institute for Biological Studies. After a previous career teaching statistics at Rowan University, she earned an M.S. in Analytics from the Georgia Institute of Technology and joined Laura Almasy's statistical genetics research group. In addition to contributing statistical computing expertise to a wide range of collaborative projects at CHOP, LiBI, and beyond, she is interested in developing new methods for analyzing genomic data.
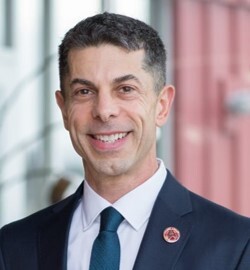
Mike Bamshad, MD (Moderator)
Editor-in-Chief of HGG Advances
Key:




