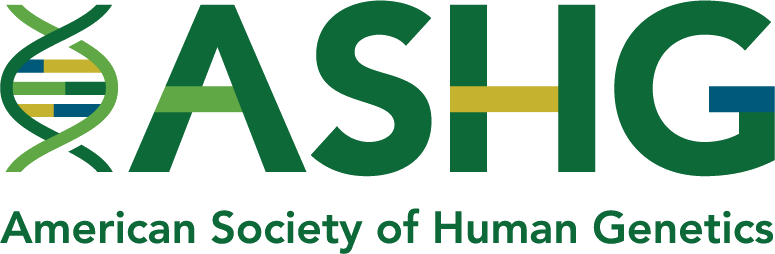
Polygenic Risk Prediction in Diverse Populations: Leveraging Ancestry and Family History
-
Register
- Regular Member - Free!
- Early Career Member - Free!
- Resident/Clinical Fellow Member - Free!
- Postdoctoral Fellow Member - Free!
- Graduate Student Member - Free!
- Undergraduate Student Member - Free!
- Emeritus Member - Free!
- Life Member - Free!
- Trainee Member - Free!
Platform sessions are abstract driven sessions with 6 talks per session. These talks are 10 minutes in length and are cross-topical in nature to represent the broad discipline our field of genetics and genomics represent. After each talk, there will be a 5-minute Q&A with each speaker. For information on each individual session, please view the "Details" tab.
Recorded session from the 2021 virtual meeting.
Key:





Local ancestry allows for improved genomic prediction in underrepresented and admixed populations
Due to the paucity of methodological and computational approaches that account for their genomic complexity, admixed populations are systematically excluded from statistical genomic studies. Admixed populations make up more than a third of the US populace but are severely underrepresented in biomedical research which may contribute to health disparities. To reap the full benefits from the ongoing efforts to collect samples from underrepresented populations and from existing mixed ancestry cohorts, tools facilitating the well-calibrated research of admixed peoples are urgently needed.
We recently developed a local ancestry aware GWAS model, Tractor, which corrects for fine-scale population structure at the genotype level, often boosts locus discovery power, and produces ancestry-specific effect size estimates and p values. Using Tractor summary statistics from African ancestry (AFR) tracts in ~4500 admixed UK Biobank (UKB) individuals, we built polygenic risk scores (PRS) and predicted blood panel phenotypes on homogenous African ancestry UKB individuals. We benchmarked these PRS against scores created from traditional GWAS runs on 1) the same admixed cohort, 2) a large European UKB sample, and 3) a large multi-ancestry meta-analysis of continental ancestry groups from the pan-UKB project (https://pan.ukbb.broadinstitute.org/). We also tested the accuracy of several PRS models including pruning and thresholding and PRS-CSx. We find that incorporating diverse samples and ancestry-specific estimates from admixed populations results in higher prediction accuracy for homogeneous AFR individuals. The bulk of African-descent GWAS participants are currently admixed individuals of the Americas, and some underrepresented ancestries are rarely found outside of the admixed context. Thus, building models based on ancestry-specific estimates generated from the deconvolved local ancestry tracts of admixed genomes allows for better PRS performance on many diverse populations from making better use of existing collections.
We additionally highlight several loci which we find to have well-demonstrated effect size differences across ancestries, a phenomenon for which there are few prior examples in the literature. As our models are constructed off of local ancestry components from the same admixed individuals, these results hint at genetic differences rather than environmental factors, which are often tricky to disentangle. Ultimately, our work highlights how Tractor and local ancestry allow for improved population characterization and can be leveraged to advance the understanding of complex diseases across diverse cohorts.
Elizabeth G Atkinson
Baylor College of MedicinePolygenic risk prediction of obesity across the life course and in diverse populations
Polygenic risk scores (PRSs) for body mass index (BMI) that leverage the increasing genome-wide association study (GWAS) sample sizes may aid risk stratification and allow targeted prevention of obesity at an early age. We constructed ancestry-specific and trans-ancestral PRSs to predict obesity in adulthood, and examined their added value over and above easily measurable predictors of obesity during childhood and adolescence.
We calculated PRSs based on summary statistics of up to 1.2 million common variants [minor allele frequency (MAF)>1%] from the GIANT consortium’s BMI GWAS meta-analysis of up to 1.6 million individuals (72% European (EUR), 16% East Asian (EAS), 6% African (AA), 4% Hispanic (HA), 2% South Asian (SAS)). Explained variance for BMI and discrimination for obesity were examined in the UK Biobank (UKB, n=437k) and Million Veteran Program (MVP, n=101k). The best performing PRS in EUR was taken forward to the Avon Longitudinal Study of Parents and Children (ALSPAC, n=5.8k), for cross-sectional and longitudinal associations with BMI across 21 time-points from birth to age 22y. We compared the predictive performance of the PRS to that of clinically available factors (maternal education, pre-pregnancy maternal BMI, household social status).
The trans-ancestral PRS explained more of the variation in BMI than ancestry-specific PRSs in all but the EUR-populations (R2 min-max for non-EUR; UKB: 7.5-12.4% (AA/EAS); MVP: 5.7-11.1% (AA/HA); UKB-EUR (EUR-PRS): 15.8%; MVP-EUR (EUR-PRS): 13.1%). For all ancestries, maximum explained variance was roughly double that of previously published obesity PRSs. The PRSs were better at discriminating between adults with or without obesity than age, sex, or scores of genome-wide significant variants only. EUR-PRS associations with BMI were weak at birth, but increased rapidly during childhood, and remained stable from adolescence onwards (e.g., BMI-SD per PRS-SD at 13y (95%CI): 0.39 (0.37,0.42)). Consistently, longitudinal modeling of BMI trajectories using the PRS showed increasing divergence until early adolescence. When added to other factors available at birth, the PRS helped predict substantially more of BMI from 5y onwards (e.g., R2 at 5y: 13 to 18%; 11y: 11 to 21%).
The current PRSs, based on larger GWAS sample sizes, double the previously explained variance for BMI across multiple ancestries, thereby advancing the options for prognostication in populations traditionally underrepresented in genetic research. Moreover, we find that genetic predisposition to adult obesity affects childhood growth trajectories, and shows potential to improve risk stratification for obesity at an early age.
Roelof A.J. Smit
The Charles Bronfman Institute for Personalized Medicine, Icahn School of Medicine at Mount Sinai
Improving Polygenic Prediction in Ancestrally Diverse Populations
Polygenic risk scores (PRS) are less effective when ported across populations. While the scale of non-European genomic resources has been expanded in recent years, a clear attenuation of the predictive performance of PRS remains in individuals who are genetically distant from Europeans.
In order to include data from all ancestral groups to ensure more equitable delivery of genomic prediction to global populations, we developed the first principled Bayesian PRS construction method, termed PRS-CSx, that jointly models GWAS summary statistics from multiple populations to improve cross-ancestry polygenic prediction. PRS-CSx couples genetic effects across populations via a shared continuous shrinkage prior, enabling more accurate effect size estimation by sharing information between summary statistics and leveraging linkage disequilibrium (LD) diversity across discovery samples, while inheriting computational efficiency and robustness from PRS-CS.
PRS-CSx outperformed existing PRS methods across various simulations settings with different sample sizes, fractions of causal variants, and genetic correlations between populations. Using quantitative traits from biobanks, we showed that PRS-CSx substantially improved the prediction accuracy even if only a small non-European GWAS was included in the discovery data. For example, the median R2 increased by 76% for individuals of East Asian ancestry when the Biobank Japan samples (N=62K-159K) were added to the UK Biobank European samples (N=340K-360K) to train the PRS. Similarly, the median R2 increased by 22% for individuals of African ancestry when the PAGE study samples (N=20K-50K) were integrated with UK Biobank and Biobank Japan samples (400K-519K).
Furthermore, by integrating GWAS summary statistics of schizophrenia from East Asian (14K-17K cases due to leave-one-out) and European (33K cases) populations, PRS-CSx more accurately predicted schizophrenia risk in individuals of East Asian ancestry, showing 52% and 97% improvement in the liability R2 relative to PRS constructed using East Asian or European summary statistics only, and approximately doubled the prediction accuracy when compared with alternative methods that can combine multiple GWAS to make prediction.
Our method represents a much needed and critical breakthrough in PRS construction. Through joint modeling of multi-ancestry data, PRS-CSx substantially improves polygenic prediction in non-European populations. With the rapid expansion of non-European genomic resources, our method will help accelerate the equitable deployment of PRS in clinical settings and maximize its healthcare potential.
Yunfeng Ruan
Stanley Center for Psychiatric Research, Broad Institute of MIT and Harvard
A trans-ancestry polygenic test to predict severe hypercholesterolemia in diverse ancestry patients
Approximately 7% of adults have severe hypercholesterolemia (SH; untreated low density lipoprotein (LDL-C) ≥ 190 mg/dL). SH is associated with a 6-fold increased risk of cardiovascular disease, and up to 20-fold increased risk in individuals identified with monogenic Familial Hypercholesterolemia (FH)-associated variants. Despite high frequency of cholesterol screening and awareness, individuals with SH remain undertreated, with disparities in treatment and LDL-C control observed among African American (AA) populations. Only 2.5% of individuals with SH harbor a monogenic FH-associated variant, and polygenic SH accounts for 15%-30% of clinical FH, motivating the development of a polygenic test for predicting SH in diverse populations. We obtained summary statistics for validated trans-ancestry polygenic risk scores (PRS) to predict LDL-C from the Global Lipids Genetics Consortium pre-publication. The PRS were developed from a genome-wide association study of ~1.6M trans-ethnic participants, and validated in European (EU), AA, African, Hispanic or Latino (HL), South Asian and East Asian populations. We leveraged independent genotype and phenotype data from the diverse BioMe biobank in New York City. We extracted laboratory values and medications from electronic health records for adults with an age range of 18-95 from three population groups: AA, EU, and HL (other groups were excluded due to low sample size). SH cases were defined as participants with statin-adjusted maximum LDL-C ≥ 190 mg/dL and controls with statin-adjusted maximum LDL-C < 160 mg/dL (EU: 323/4810, AA: 422/3741, and HL: 539/5780). In a model that included the covariates age, sex, and the top 10 principal components, we measured PRS discrimination (via AUC) which was 0.68 (0.65-0.71), 0.70 (0.68-0.73), and 0.72 (0.70-0.74) for EU, AA, and HL respectively; and 0.67 (0.64-0.70), 0.68 (0.66-0.71), and 0.65 (0.62-0.67) for the genomic predictor alone. The effect size of the PRS was 1.97 (1.74-2.24), 2.0 (1.85-2.35), and 2.02 (1.81-2.25) odds ratio (OR) per standard deviation. We established a high-risk threshold of 3%, and found effect sizes of 4.98 (3.30-7.34), 2.99 (1.89-4.61), and 3.96 (2.74-5.64) OR compared to the 97% below the threshold. We estimated prevalence-adjusted positive vs. negative predictive values of cases being classified in the high risk group, and demonstrated 0.25 vs 0.93, 0.17 vs 0.93, and 0.21 vs 0.93. In summary, we demonstrate that a PRS for LDL-C can be leveraged to predict a 3- to 5-fold increased risk of SH in diverse populations, raising the possibility that this test could be used to identify individuals predisposed to polygenic SH.
Michael C Turchin
The Institute for Genomic Health, Icahn School of Medicine at Mount Sinai
Phenome-wide association study of polygenic risk for asthma in the UK Biobank highlights traits with shared genetic architecture and sex specific effects
Polygenic risk scores (PRSs) aggregate additive effects of genetic variants to estimate individual risks for heritable diseases and can be used clinically to inform decisions on screening, therapeutic intervention, and lifestyle modification. The aim of this study was to develop a PRS for asthma using genetic information from a large, multiethnic (ME) cohort and investigate its association with 267 phenotypes in the UK Biobank (UKB). Two asthma PRS models were developed based on European (EU) (19,954 cases, 107,715 controls) and ME (23,948 cases, 118,538 controls) summary statistics from the Trans-National Asthma Genetic Consortium meta-analysis. Posterior SNP effect size estimates were generated using a Bayesian regression framework, implemented in PRS-CS. To evaluate PRS prediction for asthma, each model was applied to white British (36,065 cases, 314,781 controls) and ME (43,109 cases, 377,061 controls) subjects from UKB using logistic regressions adjusting for sex and ancestry. The EU PRS applied to the white British cohort had the strongest association with doctor-diagnosed asthma (p=1.96x10-295, OR=1.34, 95% CI=1.32-1.35, AUC=0.582) and was most strongly associated with childhood onset asthma (COA; onset before age 12; p=1.77x10-181, OR=1.59, 95% CI=1.54-1.64, AUC=0.624). There were significant sex-by-PRS interaction effects for COA (p=0.049) and adult onset asthma (AOA; onset after age 25; p=0.048). Given the same PRS, males had a higher risk than females for COA but females had a higher risk than males for AOA. The phenome-wide association study identified significant associations between the PRS and 27 binary and 69 quantitative traits (Bonferroni p<1.87x10-4). The most significant association was with percent eosinophils (p=9.33x10-298, β=0.11), a known asthma-associated trait. Other associated traits included asthma age of onset (p=4.12x10-94) and measures of lung function (FEV1) (p=1.91x10-117). Some associations were less expected. For example, age at first live birth was negatively correlated with the PRS (p=8.57x10-15, β=-0.095) and HbA1c was positively correlated with the PRS (p=4.83x10-33, β=0.13). Sex-specific effects were observed for 5 binary and 15 quantitative traits, such as fat-free mass (p=1.71x10-6, β=0.028 in females; p=0.42, β=7.6x10-3 in males). Overall, our results suggest shared genetic architectures between asthma and a broad swath of pulmonary, cardiometabolic, anthropometric, and reproductive traits, many of which had not previously been linked to asthma and some with sex-specific effects. This research was conducted using the UK Biobank Resource under application number 44300.
Yu Lin Lee
Biological Sciences Collegiate Division, Univ Chicago
Modelling hidden genetic risk from family history for improved polygenic risk prediction
With many polygenic risk scores demonstrating research and clinical utility, it is worth questioning whether family history, a traditional genetic predictor, still provides valuable information.
Family history of complex traits may be influenced by transmitted rare pathogenic variants, intra-familial shared exposures to environmental factors, as well as a common genetic predisposition. Therefore, we propose and develop a latent factor model to quantify disease risk in excess of that captured by a common SNP-based polygenic risk score, but inferable from family history. This model enables calibration of polygenic risk scores with respect to family history without fitting regression models.
We applied our model to predict adult height for 941 children in the Avon Longitudinal Study of Parents and Children. Our predictor was able to explain ~55% of the total variance in adult height, close to the estimated heritability of height and substantially higher than ~40% captured by a polygenic risk score for height or mid-parental height alone. For nine complex diseases, including metabolic syndromes, cardiovascular diseases, neurological disorders and several types of cancer, we used our model to improve polygenic risk prediction for >400,000 White British participants in the UK Biobank. For all nine complex diseases investigated in the UK Biobank, parental disease history brought significant improvements in the discriminative power of polygenic risk prediction. For instance, combined with age and sex, our predictor achieved an area under the receiver operating characteristic curve (AUROC) of 0.734 and an area under the precision-recall curve (AUPRC) of 0.171 in identifying individuals with type 2 diabetes, exhibiting significantly stronger discriminative power than the polygenic risk score (AUROC = 0.712; AUPRC = 0.148) or the parental disease history (AUROC = 0.707; AUPRC = 0.148) alone. Comparing to using a type 2 diabetes polygenic risk score, our predictor had a net reclassification index of 3.72% in identifying 20% of the population at an elevated risk.
Taken together, our work showcases an innovative paradigm for risk calculation, and supports the utility of incorporating family history into polygenic risk score-based genetic risk prediction models.
Tianyuan Lu
McGill University