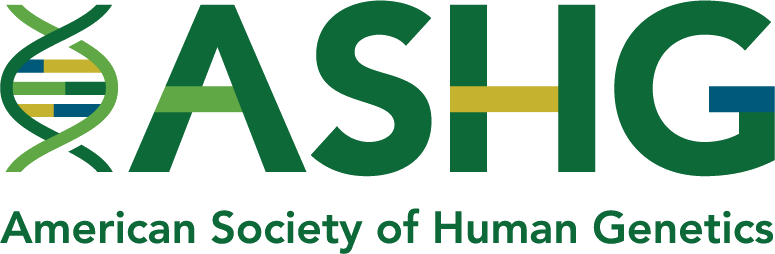
Computational Methods for Causal Variant Prioritization
-
Register
- Regular Member - $150
- Early Career Member - $75
- Resident/Clinical Fellow Member - Free!
- Postdoctoral Fellow Member - Free!
- Graduate Student Member - Free!
- Undergraduate Student Member - Free!
- Emeritus Member - Free!
- Life Member - $150
- Nonmember - $300
- Trainee Member - Free!
This session showcases state-of-the-art methods for prioritizing causal risk variants in complex trait genetic studies.
Learning Objectives:
1. Identify genetic variants associated with transcription factor binding in liver.
2. Examine using machine learning to predict and prioritize the effects of non-coding variants on diseases, leveraging functional annotations and GWAS data to identify causal variants and gain biological insights.
3. Manage robustness of summary statistics-based methods under LD mismatch.
4. Evaluate whether current genome language and other deep learning models can help pinpoint causal variants in statistical fine-mapping
Please note: This item is only available as part of the ASHG 2024 Annual Meeting Digital Pass.
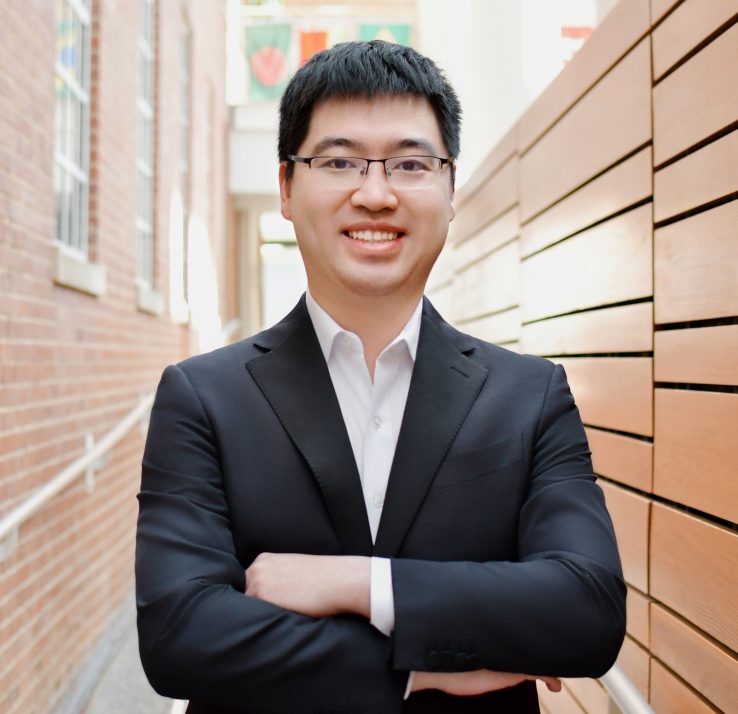
Xihao Li, PhD (Moderator)
Assistant Professor
UNC-Chapel Hill
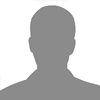
Arbel Harpak, PhD (Moderator)
Principal Investigator at the Harpak Lab
University of Texas at Austin
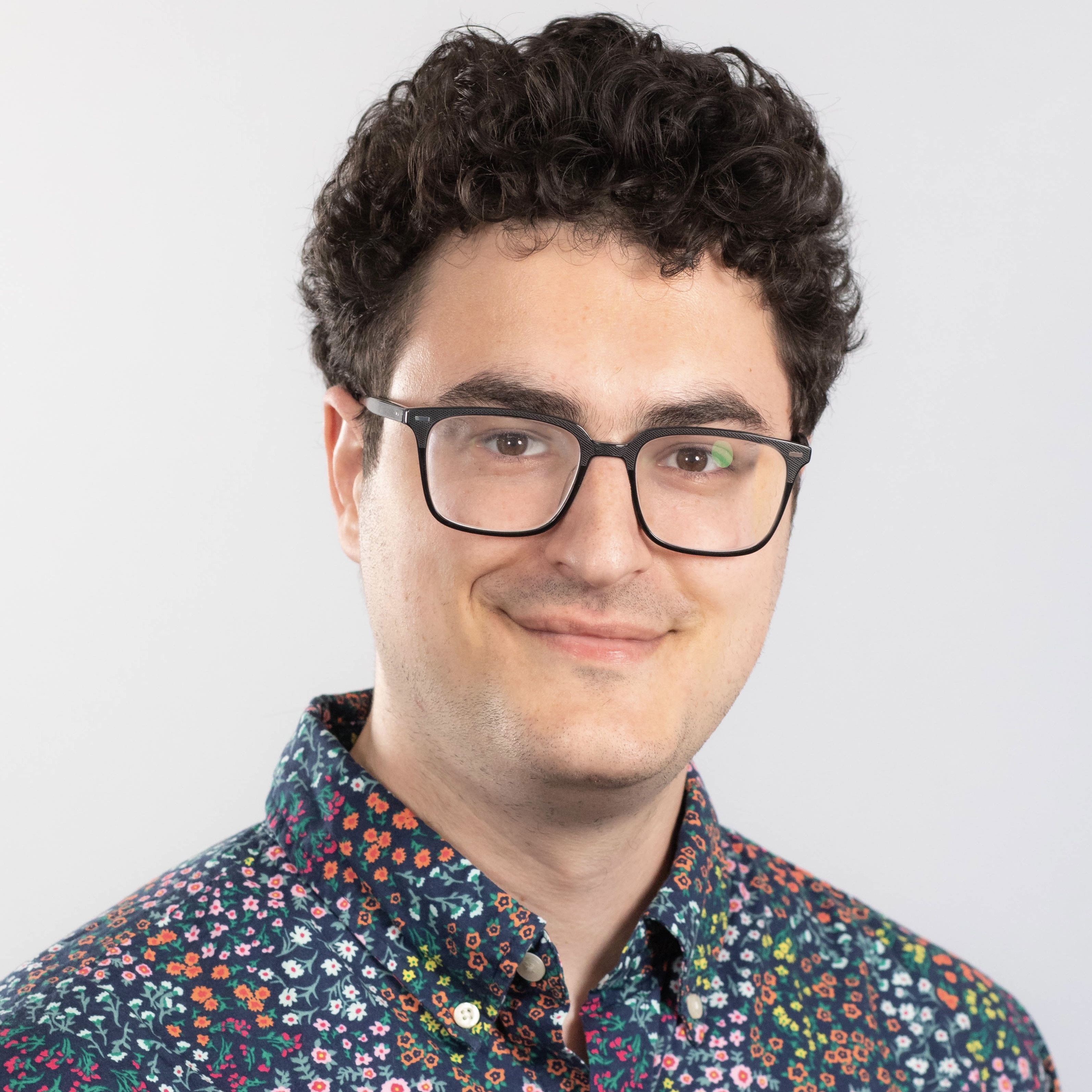
Max Dudek, BS
PhD Candidate
University of Pennsylvania
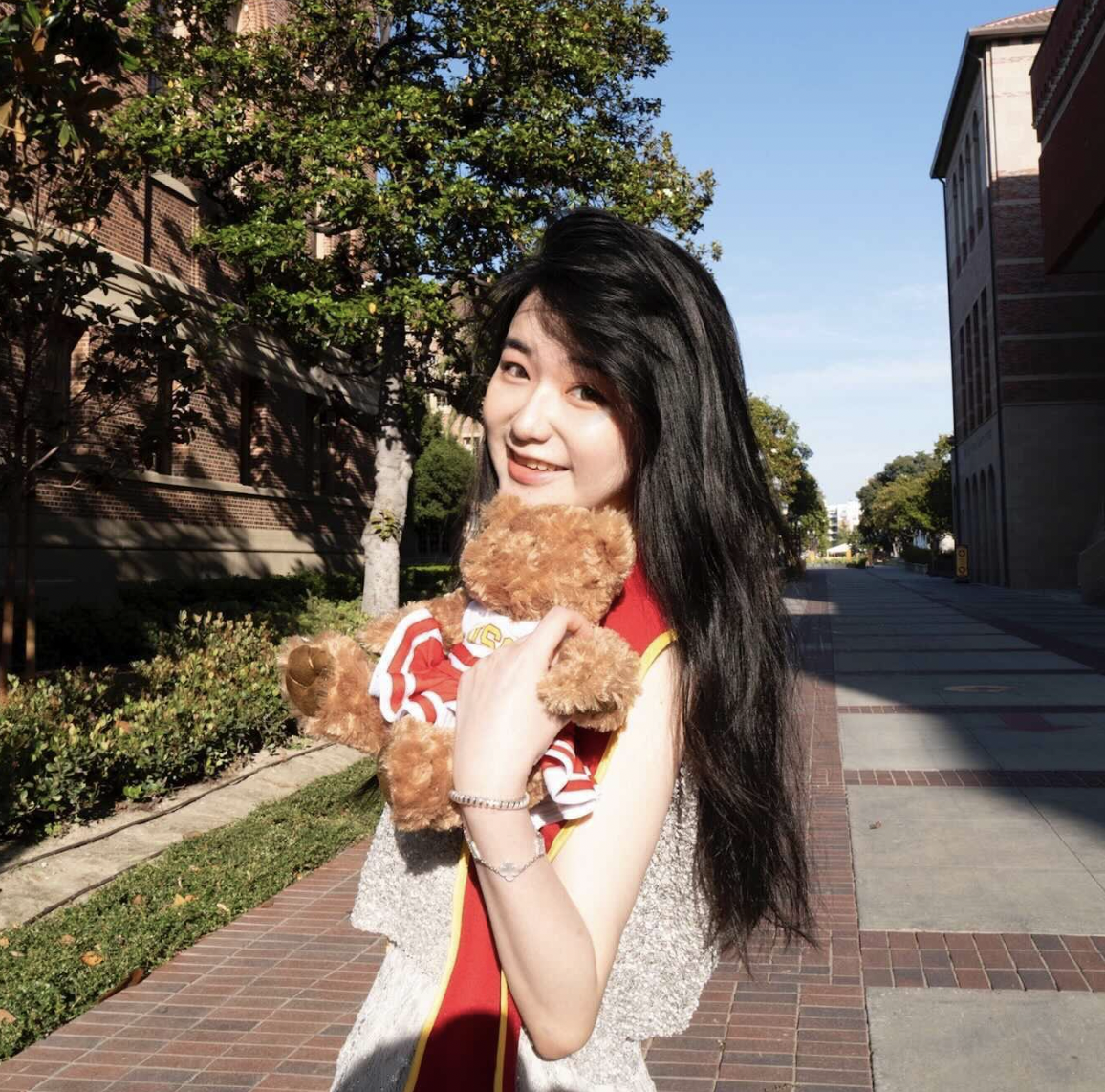
Siliangyu Cheng, MS
PhD Student
Steven Gazal Lab
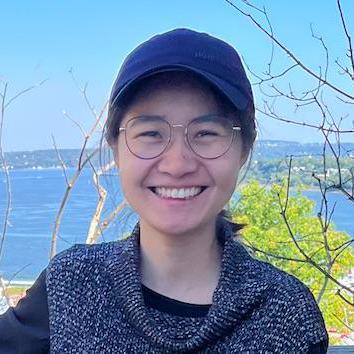
Wenmin Zhang, PhD
Postdoc at Lettre Lab
Montreal Heart Institute
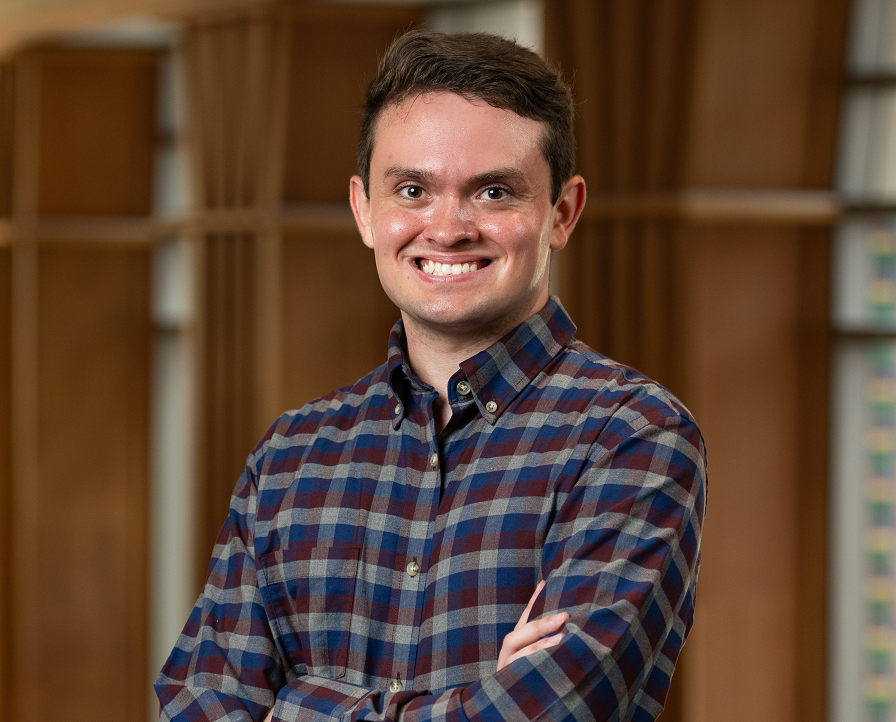